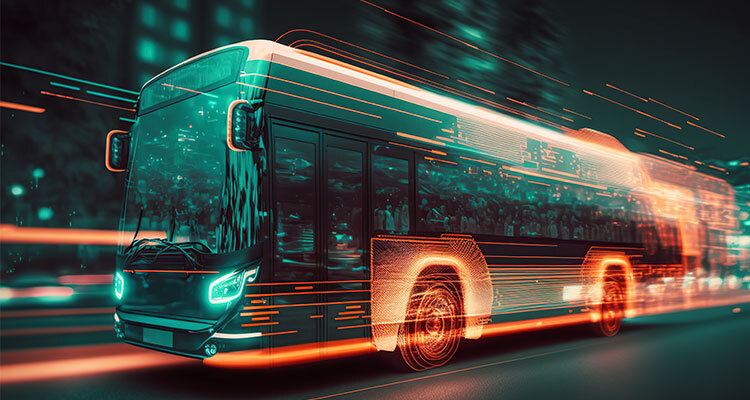
Driving a new future of public transport, one sensor at a time
The path to electrifying all vehicles comes with its fair share of challenges. With costs rising in various sectors, apprehensions are growing over the substantial investments needed to facilitate the UK’s transition to electric vehicles (EVs). To make things more complicated, the government pushed the ban on selling diesel and petrol cars back to 2035, adding to confusion and uncertainty for business planning.
Whilst switching to EV batteries would help drive lower maintenance costs, the lack of data available about battery performance is a key challenge for adoption, according to a recent report by Thatcham Research. With the inability to repair or evaluate slightly damaged battery packs after accidents – and the fact that batteries constitute approximately 40 percent of the total cost of an electric vehicle – insurers are often compelled to declare low-mileage vehicles total losses, even after minor accidents. This leads to higher premiums, thus negating the advantages of electric vehicles for consumers. The problem amplifies when it comes to managing entire fleets of electric vehicles, as the scarcity of operational data further complicates the management of maintenance and operations.
Therefore, incorporating artificial intelligence (AI) into the public transport industry is paramount in addressing these concerns. AI can facilitate obtaining real-time, actionable data insights on electric vehicle fleets. This allows operators to plan for a seamless, efficient and cost-effective service that achieves the lowest possible cost-per-mile and accelerates the achievement of cost neutrality for EV buses.
A new approach to vehicle maintenance
By utilizing vehicle data to accurately predict the remaining useful life (RUL) of components, fleet operators can safely extend the longevity of parts and systems, making vehicle servicing more predictable and less costly. Employing AI to offer real-time, actionable insights into an electric bus’ internal health enables maintenance managers to remotely diagnose malfunctions without taking the vehicle off the road for a physical examination.
Many of the largest transport operators are already equipping their vehicles with this technology. The vehicle sensor data is collected and sent back to the servers, where advanced AI and machine learning algorithms analyze it and transform it into actionable insights. This significantly reduces expenses, as dispatched engineers can assess faults beforehand and determine the most appropriate course of action. Vehicle servicing can be optimally scheduled, maximizing road time, preventing unforeseen breakdowns, and extending the operational life of components. As a result, electric buses remain on the road longer, breakdowns occur less frequently, and servicing schedules are optimized to minimize costly downtime and service disruption.
Fleets that are running fully electric operations are using a pattern-recognition and modelling analysis of the main breakdowns such as HV battery cell imbalance or diesel heater faults, leading to averted breakdowns, minimized unplanned downtime, and a better allocation of resources.
When it comes to EV batteries, public transport managers must consider battery pack degradation over time and its impact on range. Environmental factors like weather, and unpredictable variables like traffic that influence the distance a bus can travel on a single charge, are making it difficult for transport providers to efficiently plan their EV operations. For instance, a new electric bus that can run 300 km on a single charge, due to progressive battery degradation, may in a few years need an extra charging session to cover the same distance. Using advanced data analytics and machine learning to combine battery data with other range-affecting factors allows fleet managers to predict a vehicle’s remaining range accurately. Predictive battery analytics can also offer insights into the expected battery capacity loss for the coming years.
Operators cannot deliver a seamless, efficient, and cost-effective service without this understanding. Predictive battery analytics can provide a precise, comprehensive view of an EV bus’ battery health evolution, allowing for effective route planning, charging requirements, and usage optimization metrics to extend the vehicle’s lifespan. By leveraging State of Charge (SoC) and Depth of Discharge (DoD) data, fleet managers can determine if the operation profile can be adjusted to maximize battery life, thus reducing electric buses’ total cost of ownership. This analysis is crucial for a successful and profitable EV fleet deployment.
Looking into the future
The substantial initial investment required for bus operators to implement a new EV fleet can only be justified if buses remain in service for a long time rather than being in the workshop for repair or maintenance or written off after a short lifespan due to uneconomical repair procedures.
The operational context surrounding lower-emission vehicles like EVs presents uncharted territory for traditional fleet managers as it’s an entirely different skill set relating to lifecycle costs, battery maintenance, and route management. To get a return on the initial investment and successfully transition their operations to electric vehicles without adversely impacting their profits, public transport companies need a deeper strategic understanding of the EV transition – something that bus operators like Arriva and Keolis truly understand.
By investing in data that can be leveraged through AI predictive maintenance solutions, bus operators are solidifying the role of public transport in the smart cities of the future. Adopting predictive maintenance technology will not only smooth the transition to EVs but also increase public trust in public services, contributing towards a greener future.
For a list of the sources used in this article, please contact the editor.
Giorgio Sarno
www.stratioautomotive.com
Giorgio Sarno is Senior Data Scientist at Stratio Automotive. Stratio boasts the world’s number one predictive fleet maintenance platform. The company’s mission is to provide end-to-end automation to transport companies, enabling fleet operators to serve more customers, better, at a lower cost.